Vehicle Controls & Behaviors
Annual PlanVehicle-Dynamics-Conscious Real-Time Hazard Avoidance in Autonomous Ground Vehicles
Project Team
Government
Paramsothy Jayakumar, Gregory R. Hudas, U.S. Army GVSC
James Overholt, AFRL
Faculty
Tulga Ersal, University of Michigan
Industry
Mitchell Rohde, Steve M. Rohde, Quantum Signal LLC
Student
Jiechao Liu, University of Michigan
Project Summary
This project began in 2012 and was completed in 2016.
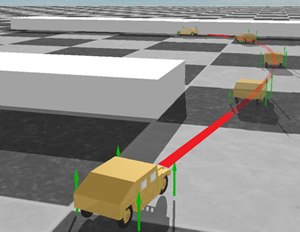
Unmanned ground vehicles (UGVs) are gaining importance and finding increased utility in both military and commercial applications. They hold a great potential for increasing mission performance, combat effectiveness, and personnel safety. UGVs span a large spectrum in terms of both platform size and mode of operation. In terms of platform size, a UGV can be a vehicle from a small ground robot to a heavy, high speed vehicle; whereas the mode of operation can range from teleoperation to full autonomy.
This scope of this work is large, fully autonomous UGVs. Within this scope, the specific problem that the work aims to address is ensuring dynamic safety during hazard avoidance maneuvers. With dynamic safety we refer to not only preventing the vehicle from running into obstacles, but also ensuring that the maneuver does not induce any stability or handling issues such as excessive side slip, tire lift off, or rollover.
Existing hazard avoidance algorithms developed for small robots do not deliver the desired performance in larger UGVs. The overarching objective of this project is to incorporate high-fidelity vehicle models into real-time hazard avoidance for large autonomous vehicle to generate dynamically safe obstacle avoidance maneuvers while minimizing the impact on travel time.
Deliverables:
A Model Predictive Control-based (MPC) Vehicle-Dynamics-Conscious Obstacle Avoidance Algorithm. The code is capable of 1) simulating control delay and sensing delay; 2) compensating control delay and sensing delay; 3) using vehicle model built in ADAMS as the plant; 4) navigating vehicle through an obstacle field that emulates the double lane change scenario, which is a scenario of interest to GVSC. The obstacle avoidance algorithm is tested extensively under various scenarios. Powertrain dynamics and soft-soil tire-terrain interaction model are incorporated in the code. Documentation of the code is also included. The current version of the code is provided to ARL, GVSC, and JPL.
Publications:
- Jiechao Liu, Paramsothy Jayakumar, James L. Overholt, Jeffrey L. Stein, Tulga Ersal. “The role of model fidelity in model predictive control based hazard avoidance in unmanned ground vehicles using LIDAR sensors”. In Proceedings of Dynamic Systems and Control Conference, paper DSCC2013-4021, 2013. doi:10.1115/DSCC2013-4021
- Jiechao Liu, Paramsothy Jayakumar, Jeffrey L. Stein, Tulga Ersal. “A Multi-Stage Optimization Formulation for MPC-Based Obstacle Avoidance in Autonomous Vehicles Using a LIDAR Sensor”, in Proceedings of ASME Dynamic Systems and Control Conference, Paper No. DSCC2014-6269, 2014. doi:10.1115/DSCC2014-6269
- Jiechao Liu, Paramsothy Jayakumar, Jeffrey L. Stein, Tulga Ersal. “An MPC Algorithm with Combined Speed and Steering Control for Obstacle Avoidance in Autonomous Ground Vehicles”, in Proceedings of ASME Dynamic Systems and Control Conference, Paper No. DSCC2015-9747, 2015. doi:10.1115/DSCC2015-9747
- Jiechao Liu, Paramsothy Jayakumar, Jeffrey L. Stein, Tulga Ersal. “A Study on Model Fidelity for Model Predictive Control-Based Obstacle Avoidance in High-Speed Autonomous Ground Vehicles”, Vehicle System Dynamics vol. 54, no. 11, pp. 1629-1650, 2016. doi:10.1080/00423114.2016.1223863
- Jiechao Liu, Paramsothy Jayakumar, Jeffrey L. Stein, Tulga Ersal. “Combined Speed and Steering Control in High-Speed Autonomous Ground Vehicles for Obstacle Avoidance Using Model Predictive Control”, IEEE Transactions on Vehicular Technology, Volume 66, Issue 10, pp. 8746 - 8763, 2017. doi:10.1109/TVT.2017.2707076
- J. Liu, P. Jayakumar, J. L. Stein and T. Ersal, “A double-worst-case formulation for improving the robustness of an MPC-based obstacle avoidance algorithm to parametric uncertainty,” 2017 American Control Conference (ACC), Seattle, WA, 2017, pp. 5562-5567. doi:10.23919/ACC.2017.7963820
- Jiechao Liu, Paramsothy Jayakumar, Jeffrey L. Stein, Tulga Ersal. “Improving the Robustness of an MPC-Based Obstacle Avoidance Algorithm to Parametric Uncertainty Using Worst-Case Scenarios”, Vehicle System Dynamics, 57:6, 874-913, doi:10.1080/00423114.2018.1492141.
Publications:
Jiechao Liu, Paramsothy Jayakumar, Jeffrey L. Stein, Tulga Ersal. “Improving the Robustness of an MPC-Based Obstacle Avoidance Algorithm to Parametric Uncertainty Using Worst-Case Scenarios”, Vehicle System Dynamics, 57:6, 874-913, doi:10.1080/00423114.2018.1492141
Jiechao Liu, Paramsothy Jayakumar, Jeffrey L. Stein, Tulga Ersal. “A Multi-Stage Optimization Formulation for MPC-Based Obstacle Avoidance in Autonomous Vehicles Using a LIDAR Sensor”, in Proceedings of ASME Dynamic Systems and Control Conference, Paper No. DSCC2014-6269, 2014. doi:10.1115/DSCC2014-6269
Jiechao Liu, Paramsothy Jayakumar, Jeffrey L. Stein, Tulga Ersal. “An MPC Algorithm with Combined Speed and Steering Control for Obstacle Avoidance in Autonomous Ground Vehicles”, in Proceedings of ASME Dynamic Systems and Control Conference, Paper No. DSCC2015-9747, 2015. doi:10.1115/DSCC2015-9747
Jiechao Liu, Paramsothy Jayakumar, Jeffrey L. Stein, Tulga Ersal. “A Study on Model Fidelity for Model Predictive Control-Based Obstacle Avoidance in High-Speed Autonomous Ground Vehicles”, Vehicle System Dynamics vol. 54, no. 11, pp. 1629-1650, 2016. doi:10.1080/00423114.2016.1223863
Jiechao Liu, Paramsothy Jayakumar, Jeffrey L. Stein, Tulga Ersal. “Combined Speed and Steering Control in High-Speed Autonomous Ground Vehicles for Obstacle Avoidance Using Model Predictive Control”, IEEE Transactions on Vehicular Technology, Volume 66, Issue 10, pp. 8746 - 8763, 2017. doi:10.1109/TVT.2017.2707076
J. Liu, P. Jayakumar, J. L. Stein and T. Ersal, “A double-worst-case formulation for improving the robustness of an MPC-based obstacle avoidance algorithm to parametric uncertainty,” 2017 American Control Conference (ACC), Seattle, WA, 2017, pp. 5562-5567. doi:10.23919/ACC.2017.7963820