Vehicle Controls & Behaviors
Annual PlanRemote Sensing Based Terrain Strength Characterization for the Next Generation NATO Reference Mobility Model Development
Project Team
Government
Paramsothy Jayakumar, U.S. Army GVSC
Andrei Abelev, Naval Research Laboratory
Vineet Gupta, Geospatial Research Laboratory, ERDC, USACE
Industry
Russ Alger, Keweenaw Research Center
Student
Jordan Ewing, Michigan Tech
Project Summary
Project started in 2018 and is ongoing.
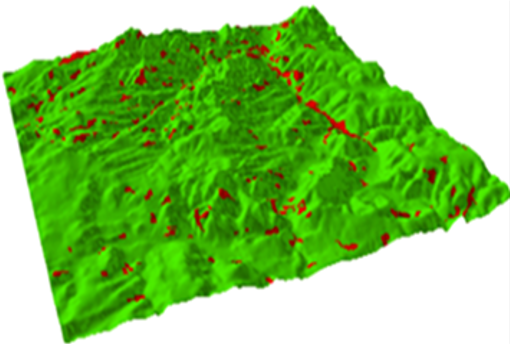
Determining the terrain strength properties is critical for achieving accurate mobility performance prediction as well as reliable operational planning using the NATO Reference Mobility Model (NRMM). The NRMM is a computer-based simulation tool that NATO forces utilize to predict the capability of a vehicle to move over specified terrain conditions. NRMM was developed and validated by U.S. Army Tank Automotive Research, Development, and Engineering Center (GVSC), and the Engineer Research and Development Center (ERDC) in the 1960s and has undergone several updates over the years. Even though NRMM is useful, it has several significant limitations. One of the major weaknesses is that it relies heavily on in-situ soil measurements, which is difficult to obtain from unknown territories and combat zones. Therefore, having the ability to remotely sense the terrain strength properties of the areas that lack in-situ soil measurement and to utilize this remotely sensed data for NRMM model is imperative for the mobility of forces in unknown territory.
The primary objective of this project is to develop remote sensing based terrain strength characterization that is suitable as an input for the NG-NRMM. To this end, the relationship between soil strength and hyperspectral and thermal remote sensing will be explored. Previous studies have shown that individually these sensing techniques are promising for strength characterization, but conclusive validation of its applicability in different terrain conditions have not been verified. The objective of this project is to combine the output from both the sensors to derive a robust estimate of terrain strength characteristics. The combined analysis will be carried out using machine learning algorithms that have shown promise to derive complex nonlinear relationship.