Intelligent Power Systems
Annual PlanComputationally-efficient 3D Models for Electric Machines
Project Team
Government
Denise Rizzo, Scott Shurin, U.S. Army GVSC
Industry
Xiao Hu, ANSYS
Arun Muley, Boeing
Jon Zeman, Gamma Technologies
Student
Yuanying Wang, University of Michigan
Project Summary
Project duration 2016-2018.
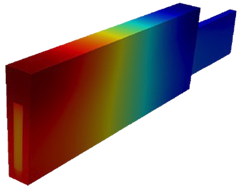
The performance of an electric machine is significantly constrained by its internal temperature. Hence, real-time knowledge of the temperatures can be very useful in determining the machine’s torque and power capability at any given instant in time, especially under severe duty cycles. The temperature information can also be used for online monitoring and protection purposes in machine design optimization, or in full-scale vehicle-level powertrain simulation, optimization, and thermal management. Therefore, it is important that vehicle-system-level design and control engineers have access to accurate computationally-efficient, physics-based modeling tools of the thermal behavior of electric machines. However, in order to be of use in these applications, the thermal models must be both accurate and computationally efficient.
The objective of this project is to develop computationally-efficient yet sufficiently-accurate electromagnetic and thermal models for electric machines which are suitable for use in vehicle design and control. These models will be created through the use of computational fluid dynamic models and model order reduction techniques. If successful, an added benefit is the ability to quickly generate and determine the performance of new machine designs through the application of scaling techniques to the developed thermal models.
We will investigate the scalability of thermal dynamics at the level of fundamental physics. With these techniques, powertrain or system designers can easily and quickly adjust the characteristics and the performance of the machine in ways that are favorable to the overall vehicle performance. For the electromagnetic portion of this work, we will extend the eigenmode-based model-order-reduction approach we have developed for thermal 3D FEA models to MQS 3D FEA models. We also seek to extend our thermal model development by creating computationally efficient models of thermal convection at the machine boundaries due to air flow.
Publications from Prior Work (closely related to current project):
- K. Zhou, A. Ivanco, Z. Filipi, H. Hofmann. “Finite-Element-Based Computationally-Efficient Scalable Electric Machine Model Suitable for Electrified Powertrain Simulation and Optimization”, IEEE Transactions on Industry Applications, vol. 51, no. 6, pp. 4435-4445, Nov.-Dec. 2015. doi:10.1109/TIA.2015.2451094
- K. Zhou, J. Pries, H. Hofmann. “Computationally-Efficient 3D Finite-Element-Based Dynamic Thermal Models of Electric Machines”, IEEE Transactions on Transportation Electrification, vol. 1, no. 2, pp. 138-149, Aug. 2015. doi:10.1109/TTE.2015.2456429
- J. Pries and H. Hofmann. “Steady-State Algorithms for Nonlinear Time-Periodic Magnetic Diffusion Problems using Diagonally-Implicit Runge-Kutta Methods”, IEEE Transactions on Magnetics, vol. 51, no. 4, pp. 1-12, April 2015. doi:10.1109/TMAG.2014.2344005