Systems of Systems & Integration
Annual PlanAutonomously Learning Mobility Limits
Project Team
Principal Investigator
Ilya Kolmanovsky, University of Michigan Anouck Girard, University of MichiganGovernment
Denise Rizzo, Ian Stranaly, U.S. Army GVSC
Industry
Ken Butts, Toyota Motor Engineering & Manufacturing North America, Inc. (TEMA)
Student
Kaiwen Liu, Nan Li, Manuel Lanchares-Prieto, University of Michigan
Project Summary
Projected started in 2018 and is ongoing.
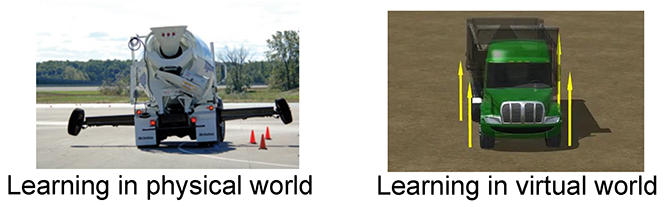
As powertrain systems and ground vehicles operate in uncertain environment and are subject to manufacturing variability, aging, degradation and damage in adversarial scenarios, the constraint boundaries can often be uncertain and maneuvers that can cause constraint violation, i.e., violations of thermal, power, and traction limits, may be a priori unknown. A common practice is then to operate these systems and vehicles conservatively to avoid constraint violation in the worst case “tolerance stack up” scenario. Such conservative operation ensures safety but can severely limit vehicle performance and mobility.
A novel, emerging approach to address the above performance-robustness tradeoff is to integrate prediction and learning/adaptation. In non-safety critical cases, where occasional constraint violation is undesirable but does not lead to catastrophic consequences, such systems may initially operate with constraint violations and learn over time to avoid them by less aggressive maneuvering. In safety critical cases, in which constraint violation is not permitted during learning, such systems will initially operate conservatively, and then improve their performance as they learn more about constraint boundaries and maneuvers that approach closely the constraint boundary. In military applications, by integrating prediction and learning/adaptation, mission feasibility and completion time can be positively impacted.
This research project develops and demonstrates novel algorithms and supporting theory for autonomously learning to operate powertrain systems and ground vehicles safely and non-conservatively. Safety refers to the operation without violation of critical limits (imposed as pointwise-in-time state and control constraints), while non-conservatism refers to the ability to follow specified commands (set-points, way-points, etc.) fast and with small tracking errors thereby achieving high performance.
Publications and Presentations
- K. Liu, N. Li, D. Rizzo, E. Garone, I. Kolmanovsky, and A. Girard, “Model-free learning to avoid constraint violations: An explicit reference governor approach,” 2019 American Control Conference, submitted, under review.